Is there a Human Machine Interface for Predictive Maintenance?
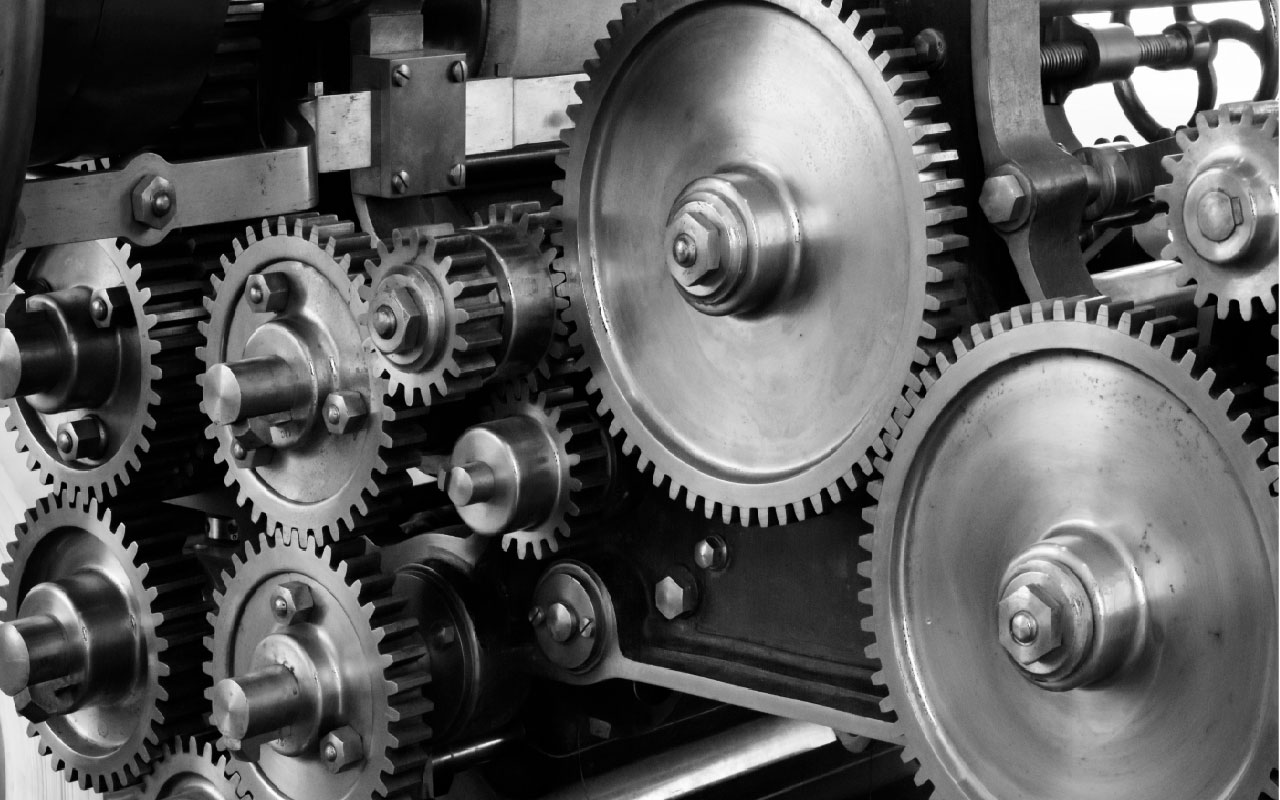
Today, companies in many heavy industries such as manufacturing, power generation, oil & gas, renewables, metals, chemicals, and mining are applying predictive maintenance (PdM) techniques to their multi-million dollar global operations to reduce the costs and downtime associated with unexpected critical machine failure and damage.
In many ways, a doctor-patient relationship is a good analogy to the technician-machine relationship in terms of trying to understand the significance of an observed condition. Imagine a patient’s body temperature is higher than normal. This could be caused by several things – some normal (e.g. strenuous exercise) and some that indicate sickness (e.g. fever) that should be treated.
Once the higher body temperature is identified, it makes sense to then look at additional factors to reach a clear prognosis. In this case, body temperature is what I call a good “leading signal” in the case of monitoring the human body. Leading signals are also a good solution for PdM in industrial machines, but before we get to that, let’s step back and look at the problem for context.
Industry 4.0 and the evolution of Predictive Maintenance
Industry 4.0 or what has been called the “smart factory” is starting to mature with massive projected investments in cyber-physical systems, cloud computing, and the Internet of Things. Today, global demand for industrial automation systems is on the rise as manufacturing companies seek ways to lower production costs while improving quality. This demand is also fueling major investments in industrial automation software and specifically AI-powered predictive analytics, a market that will reach $18.5 billion by 2021 according to Research and Markets.
However, in many cases, Predictive Maintenance, which stems from predictive analytics, is still performed on a periodic basis by technicians going from machine to machine and taking manual condition samples. For example, a technician may take vibration readings on a machine to detect deterioration in bearing condition. This method is still better than the old style of preventative maintenance, but just like industrial automation itself is evolving, new advancements in predictive analytics and deep learning are emerging where data is continuously collected and analyzed in real time. While fully automated PdM seems ideal in terms of efficiency, this scenario isn’t without its own challenges.
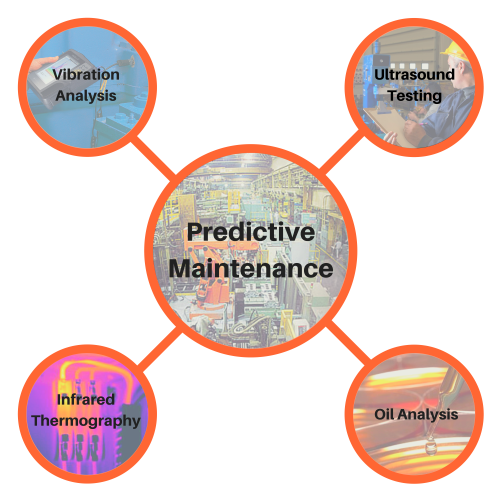
Challenges and obstacles with automated Predictive Maintenance
Companies adopting automated Predictive Maintenance are leveraging IIoT solutions that log massive amounts of data and apply thresholding methods or machine learning to identify anomalies.The challenge here is that once these data collection systems are implemented at scale, there is a resulting tsunami of data that makes the task of finding actionable insights for meaningful PdM difficult. This is the problem inherent in all situations where ‘big data’ is prevalent. AI is increasingly being used to solve the big data problem, but even such learning AI algorithms rely on context and guidance to produce meaningful insights.
When anomalies are detected through automated Predictive Maintenance – even when augmented with certain IIoT solutions that employ machine learning, it’s still hard to understand the cause and significance of the anomaly because the samples weren’t collected by an experte. Detecting anomalies with the traditional manual condition monitoring techniques actually helped the technician reach a specific prognosis. IIoT solutions aim to improve the communication flow between machines and professionals. However,with automated PdM, a part of the intimate relationship between the technician and machine is broken, which makes understanding anomalies detected remotely very difficult.
“Leading signals” as a viable solution
A proposed solution to these challenges that doesn’t revert to manual diagnostics and that reaps the benefits of automation is to focus on a limited number of “leading signals” while looking for anomalies that signify problems with the machine’s condition. The point with leading signals is to look at the big picture first with clear signals that we easily understand and alert us that something isn’t right. Conversely, if we try to ingest all of the available data at once, we drown in a sea of false positive alerts about minor anomalies that don’t need attention.
Once an anomaly is identified with a leading signal and is easily understood as a meaningful anomaly, then additional insights and data can be analyzed from other collected signals to facilitate remote diagnostics and root cause processes.
Leading signals are best when they’re something that humans easily understand and instantly trigger our intuition (like a patient’s body temperature). When these signals are humanly relatable through senses like hearing, vision, or touch, the process of validating the significance of anomalies is much easier, faster for humans who already face cognitive overload with information from automated systems.
The role of sound in automated PdM
For industrial machine PdM there are many kinds of leading signals that humans can easily understand. Again, PdM to date has been contact-based primarily (going from machine to machine with a sensor) and detects anomalies with leading signals like vibration, temperature, and power consumption. Vibration for example is a good leading signal, however in the case automated PdM, it’s much more dependent on the location of the sensor, so to fully cover a large and complex machine, several sensors will be required. This in turn contributes to the data deluge problem.
In the case of machine PdM, sound is an excellent leading signal to identify anomalies because it’s easy for humans to understand – even if they are not physically near the machine. An anomaly in the sound can be localized to its source after it’s identified. Therefore the coverage of the machine is much better even with a single sensor, and the signal represents the normal or abnormal condition of the entire machine.
Sound as a leading signal for automated PdM enables detection and classification of a wide range of mechanical phenomena, often sooner than other sensing methods. This is due to the simple fact that moving parts – whether solid, liquid or gas – produce a unique sound pattern, and when something in that movement changes, even slightly, the sound produced changes too. Take for example a mechanical bearing that’s subjected to extreme dynamic stress. When the first crack appears, the bearing will produce a unique ultrasonic pattern that indicates the trouble ahead.
Conclusion
As new technologies come to market, automated PdM will continue to evolve. But the challenges of harvesting insights from data and keeping humans and machines connected for the best diagnostics will never change. As with all big data problems, focusing on small amounts of understandable data and then widening the scope of analysis from there is the best solution. Starting with leading signals – those things that humans instantly recognize – is the key to optimizing automated PdM, and sound is a logical signal that helps address these challenges in significant and measurable ways.
This article was orginially published at IIoT World and writen by Amnon Shenfeld, a software engineer by training and an experienced hands-on software developer passionate about creating disruptive software applications. Amnon is currently CEO and co-founder of 3DSignals, a company that pioneered the application of sound-based predictive maintenance for industrial and manufacturing equipment.